Learning OUTCOMES
- Based on the study design, determine what types of conclusions are appropriate.
We now focus more closely on studies that investigate a relationship between two variables. In these types of studies, one variable is the explanatory variable, and the other is the response variable. To establish a cause-and-effect relationship, we want to make sure the explanatory variable is the only thing that impacts the response variable. We therefore get rid of all other factors that might affect the response. Then we manipulate the explanatory variable. Our goal is to see if it really does affect the response.
In an observational study, researchers may take steps to reduce the influence of these other factors on the response. But it is difficult in an observational study to get rid of all the factors that may have an influence. In addition, the researchers do not manipulate the explanatory variable to see if it affects the response. They just collect data and look for an association between the two variables. For these reasons, observational studies do not give convincing evidence of a cause-and-effect relationship.
In an experiment, researchers use a variety of techniques to eliminate the influence of these other factors. Then they manipulate the explanatory variable to see if it affects the response. For this reason, experiments give the strongest evidence for a cause-and-effect relationship.
Example
Hormone Replacement Therapy
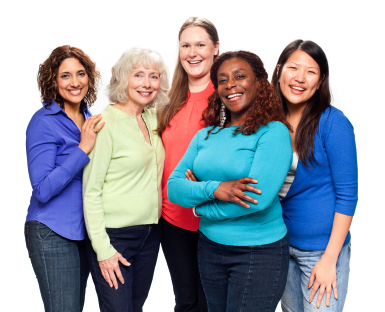
When women go through menopause, the production of hormones in their bodies changes. The hormonal changes can cause a variety of symptoms that may be reduced by hormone replacement therapy. In the 1980s, hormone replacement therapy was common in the United States.
In the early 1990s, observational studies suggested that hormone replacement therapy had additional benefits, including a reduction in the risk of heart disease. In these observational studies, researchers compared women who took hormones to those who did not take hormones. Health records showed that women taking hormones after menopause had a lower incidence of heart disease. But women who take hormones are different from other women. They tend to be richer and more educated, to have better nutrition, and to visit the doctor more frequently. These women have many habits and advantages that contribute to good health, so it is not surprising that they have fewer heart attacks. But can we conclude from these studies that the hormones caused the reduction in heart attacks? No. The results are confounded by the influence of these other factors.
In 2002, the Women’s Health Initiative sponsored a large-scale, well-designed experiment to study the health implications of hormone replacement therapy. In this experiment, researchers randomly assigned over 16,000 women to one of two treatments. One group took hormones. The other group took a placebo. A placebo is a pill with no active ingredients that looks like the hormone pill. The experiment was double-blind. Blind means that women did not know if they were receiving hormones or the placebo. Double-blind means that the information was coded, so researchers administering the pills did not know which treatment the women received. After 5 years, the group taking hormones had a higher incidence of heart disease and breast cancer. This is exactly the opposite result from the result found in the observational studies! In fact, the differences were so significant that the researchers ended the experiment early. The National Institutes of Health declared that the observational studies were wrong. Hormone replacement therapy to treat menopausal symptoms is now rarely used.
What’s the Main Point?
An observational study can provide evidence of a link or an association between two variables. But other factors may also influence the results. These other factors are called confounding variables. The influence of confounding variables on the response variable is one of the reasons that an observational study gives weak, and potentially misleading, evidence of a cause-and-effect relationship. A well-designed experiment takes steps to eliminate the effects of confounding variables, including random assignment of people to treatment groups, use of a placebo, or blind conditions. Using these precautions, a well-designed experiment provides convincing evidence of cause-and-effect.
Try It